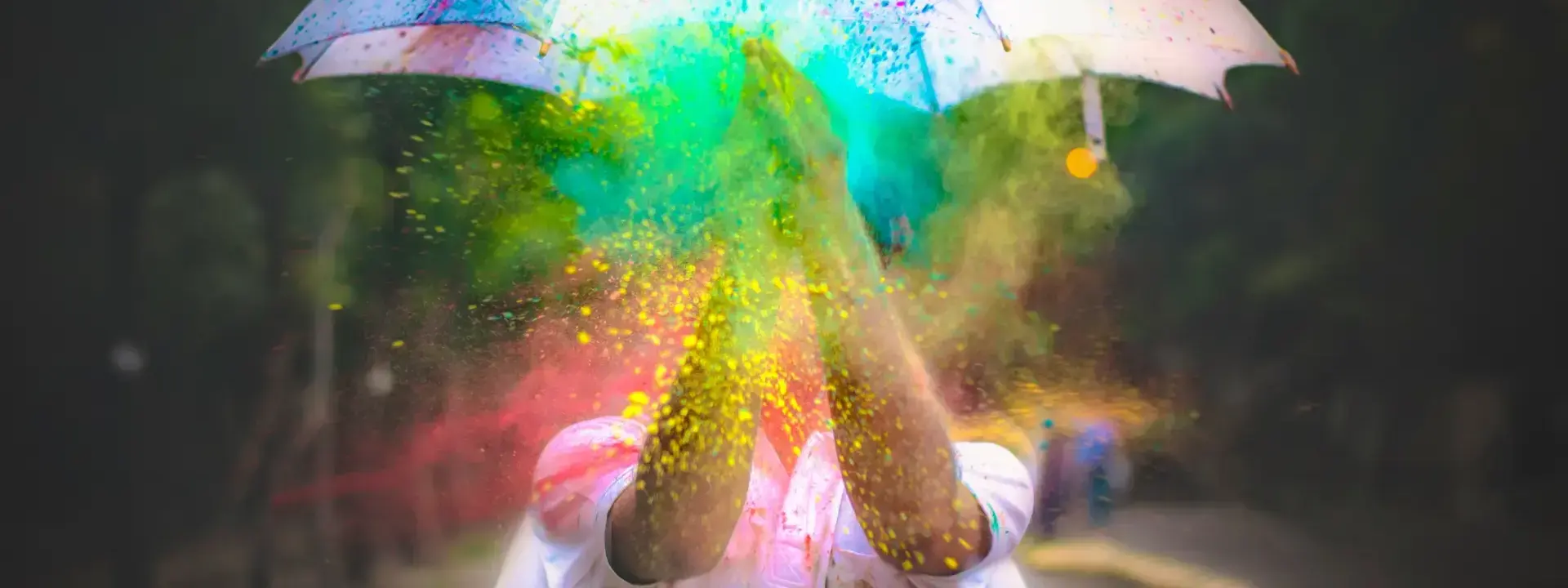
Understanding and Measuring Algorithmic Bias vs. Human Bias vs. Subconscious Bias
Introduction
In an increasingly digitized world where algorithms govern everything from job applications to credit approvals, the issue of bias has taken center stage. But what exactly do we mean by bias, and how does it manifest differently in algorithms, humans, and our subconscious? In this blog, we'll delve into the complexities of bias, explore how it manifests in various forms, and discuss the challenges and methods of measuring bias in algorithms, human decision-making, and subconscious biases.
Defining Bias:
Bias, at its core, refers to the systematic favoritism or prejudice towards or against particular groups, individuals, or ideas. It can manifest consciously or unconsciously, impacting decisions, actions, and outcomes. Bias can stem from various sources, including cultural norms, personal experiences, societal structures, and cognitive processes.
Human Bias:
Human bias refers to the inherent prejudices or preferences that individuals possess based on their personal experiences, beliefs, and socialization. It can influence decision-making in various contexts, such as hiring practices, criminal justice proceedings, and interpersonal interactions. Human bias often arises from stereotypes, implicit associations, and ingrained attitudes towards certain groups.
Examples of human bias include:
- Confirmation Bias: The tendency to interpret information in a way that confirms preexisting beliefs or assumptions.
- Stereotyping: Generalizing characteristics or traits to an entire group based on limited information or biases.
- In-group Bias: Favoring individuals who belong to the same social, cultural, or identity group as oneself.
- Affinity Bias: Preferring individuals who share similar backgrounds, interests, or experiences.
Subconscious Bias:
Subconscious bias, also known as implicit bias, refers to the automatic associations and attitudes that individuals hold towards certain groups or concepts, often without conscious awareness. These biases are deeply ingrained and can influence perceptions, judgments, and behaviors, even among well-intentioned individuals. Subconscious biases develop through exposure to societal stereotypes and cultural messaging, shaping our perceptions and responses on a subconscious level.
Examples of subconscious bias include:
- Race Bias: Unconscious associations between race and attributes such as intelligence, trustworthiness, or competence.
- Gender Bias: Automatic preferences or stereotypes based on gender, influencing evaluations and decisions.
- Age Bias: Unconscious attitudes towards individuals of different age groups, impacting perceptions of competence or relevance.
- Appearance Bias: Implicit judgments based on physical appearance, such as attractiveness or grooming.
Algorithmic Bias:
Algorithmic bias refers to the systemic discrimination or unfairness present in automated decision-making systems, often resulting from biased data, flawed algorithms, or biased design choices. Algorithms, like humans, can perpetuate and even exacerbate societal biases, leading to discriminatory outcomes in areas such as hiring, lending, and criminal justice.
Examples of algorithmic bias include:
- Data Bias: Biases present in the training data used to develop algorithms, leading to skewed or incomplete representations of certain groups.
- Algorithmic Fairness: Differential treatment of individuals or groups by algorithms, resulting in disparate impacts or outcomes.
- Feedback Loops: Algorithms that reinforce existing biases through self-reinforcing feedback loops, exacerbating inequality and discrimination.
- Lack of Transparency: Black-box algorithms that obscure the decision-making process, making it challenging to identify and address biases.
Measuring Bias:
Measuring bias in algorithms, human decision-making, and subconscious biases poses significant challenges due to their complex and multifaceted nature. However, researchers have developed various methods and techniques to assess and mitigate bias in each of these domains.
Algorithmic Bias Measurement:
- Bias Audits: Evaluating algorithms for disparate impacts on different demographic groups through statistical analysis of outcomes.
- Fairness Metrics: Developing quantitative measures of fairness and equity to assess algorithmic decision-making.
- Data Analysis: Scrutinizing training data for biases and underrepresented groups, and correcting imbalances through data preprocessing techniques.
- Algorithmic Transparency: Promoting transparency and accountability in algorithmic decision-making processes to identify and mitigate biases.
Human Bias Measurement:
- Implicit Association Tests (IAT): Assessing subconscious biases through reaction time tests that measure associations between concepts and attributes.
- Observational Studies: Analyzing real-world decision-making processes to identify patterns of bias in various contexts.
- Diversity Training: Implementing training programs to raise awareness of biases and promote inclusive decision-making practices.
- Feedback Mechanisms: Soliciting feedback from diverse stakeholders to identify and address biases in decision-making processes.
Subconscious Bias Measurement:
- Implicit Association Tests (IAT): Assessing subconscious biases through reaction time tests that measure associations between concepts and attributes.
- Neuroscientific Studies: Using brain imaging techniques to investigate the neural mechanisms underlying subconscious biases.
- Behavioral Experiments: Conducting controlled experiments to measure the impact of subconscious biases on decision-making and behavior.
- Intervention Strategies: Implementing interventions such as stereotype replacement or perspective-taking exercises to reduce subconscious biases.
Conclusion:
Bias, whether algorithmic, human, or subconscious, poses significant challenges to fairness, equity, and social justice. Understanding the differences between these forms of bias and developing effective measurement techniques are crucial steps towards addressing bias in decision-making processes. By fostering transparency, accountability, and inclusivity, we can work towards creating more equitable systems and societies, where bias has minimal influence on outcomes and opportunities for all individuals and groups.
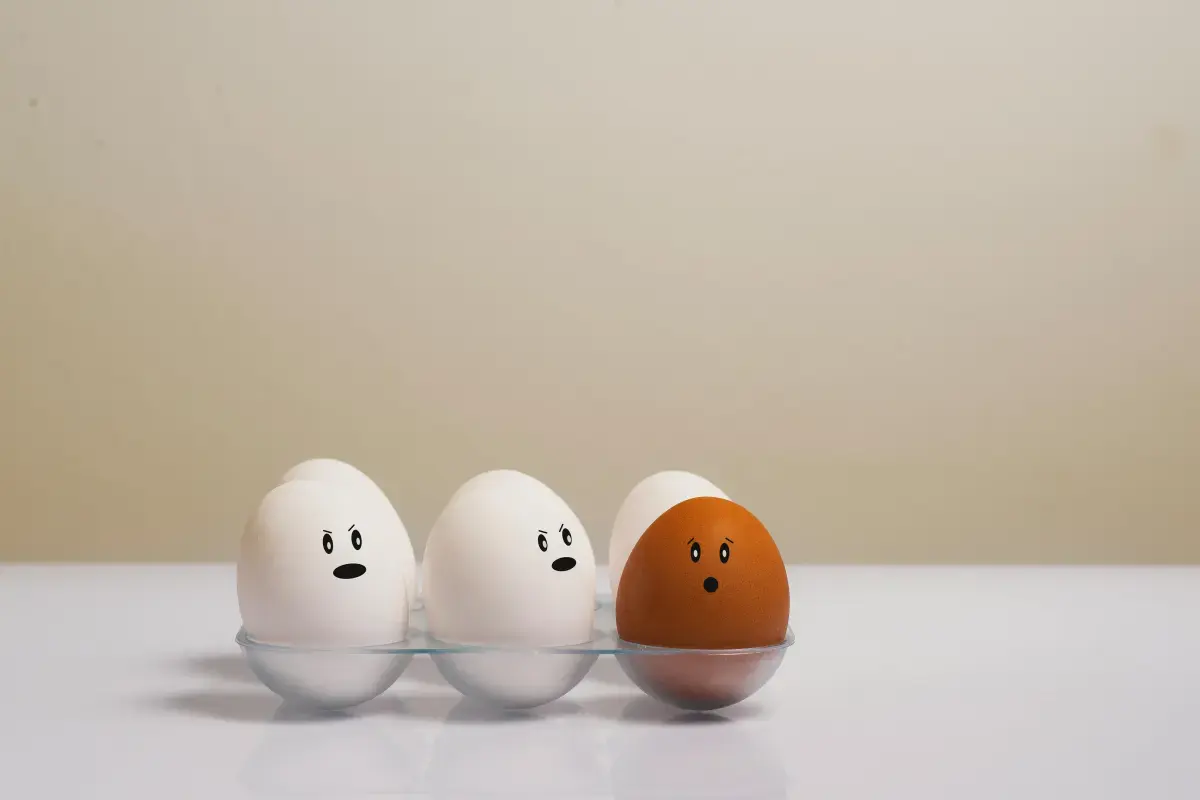